You get what you measure: New NLU benchmarks for few-shot learning and robustness evaluation
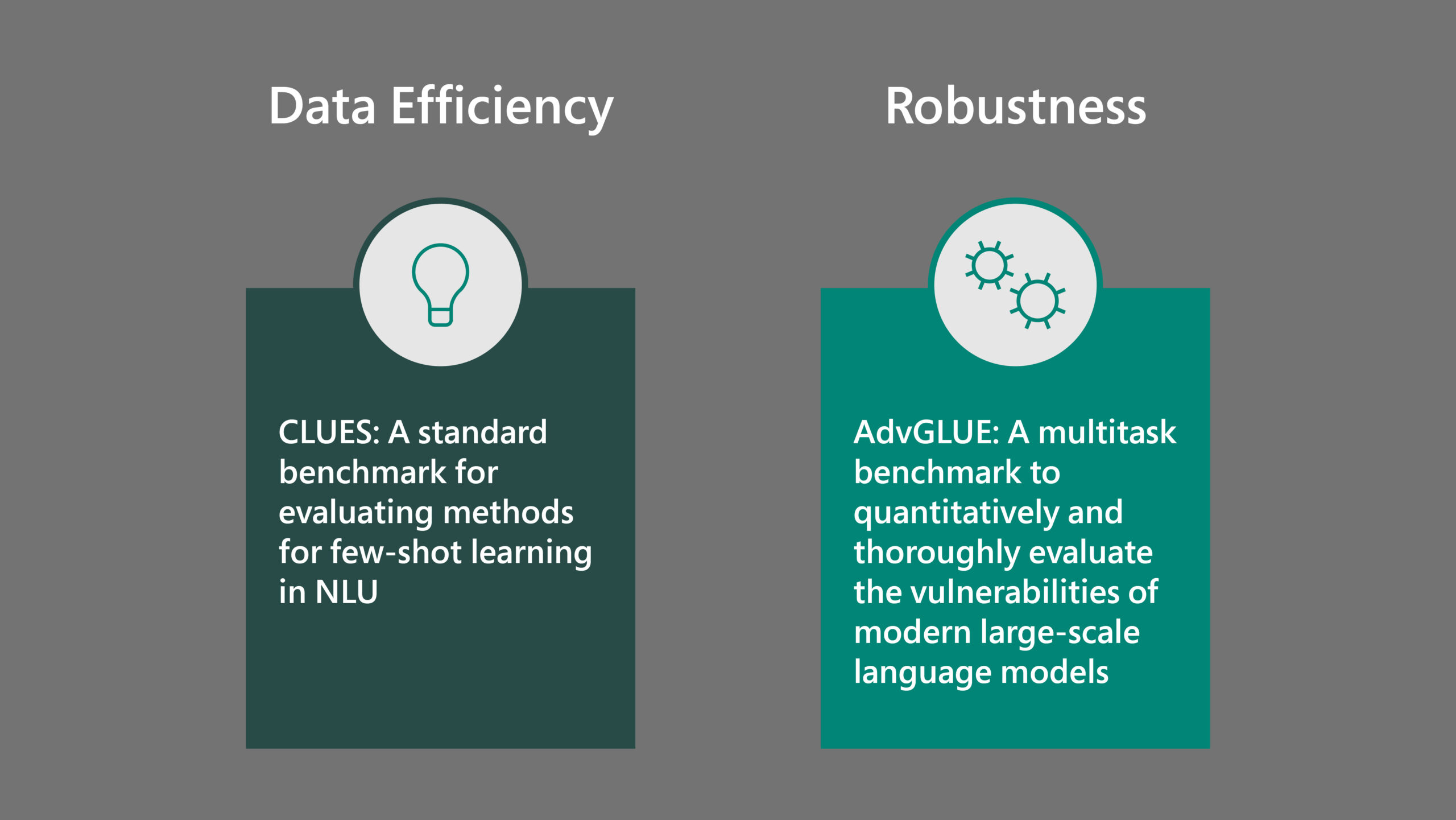
Recent progress in natural language understanding (NLU) has been driven in part by the availability of large-scale benchmarks that provide an environment for researchers to test and measure the performance of AI models. Most of these benchmarks are designed for academic settings–typically datasets that feature independent and identically distributed (IID) training, validation, and testing sections drawn from data that have been collected or annotated by crowdsourcing.
However, increasing evidence shows that AI models that achieve human-level performance on academic benchmarks may underperform in real-world settings where a) task-specific labels are unavailable for model training and b) the dataset contains various adversarial examples. Ironically, models that reached human-level performance in academic settings highlight the limitation