Why Aren’t My Results As Good As I Thought? You’re Probably Overfitting
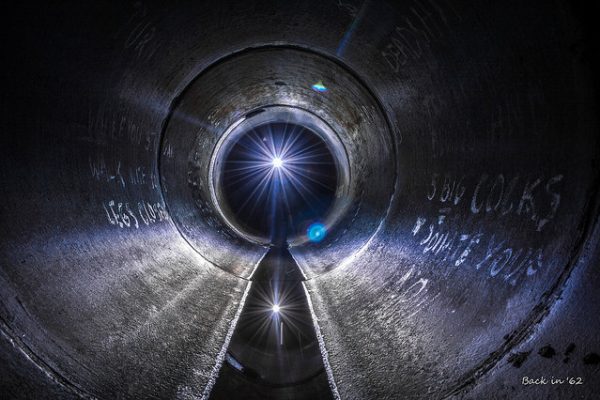
Last Updated on August 15, 2020
We all know the satisfaction of running an analysis and seeing the results come back the way we want them to: 80% accuracy; 85%; 90%?
The temptation is strong just to turn to the Results section of the report we’re writing, and put the numbers in. But wait: as always, it’s not that straightforward.
Succumbing to this particular temptation could undermine the impact of otherwise completely valid analysis.
With most machine learning algorithms it’s really important to think about how those results were generated: not just the algorithm, but the dataset and how it’s used can have significant effects on the results obtained. Complex algorithms applied to too-small datasets can lead to overfitting, leading to misleadingly good results.

Light at the end of the tunnel
Photo by darkday, some rights reserved
What is Overfitting?
Overfitting occurs when a machine learning algorithm, such as a classifier, identifies not only the signal in a dataset, but the noise as well. All datasets are noisy. The
To finish reading, please visit source site