Training Stable Diffusion with Dreambooth
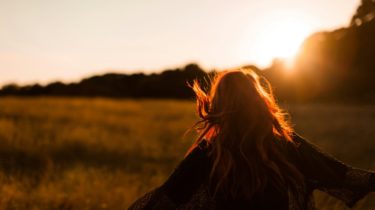
Stable Diffusion is trained on LAION-5B, a large-scale dataset comprising billions of general image-text pairs. However, it falls short of comprehending specific subjects and their generation in various contexts (often blurry, obscure, or nonsensical). To address this problem, fine-tuning the model for specific use cases becomes crucial. There are two important fine-tuning techniques for stable Diffusion:
- Textual inversion: This technique focuses on retraining the text embeddings of a model to inject a word as a subject.
- DreamBooth: Unlike textual inversion, DreamBooth involves the retraining of the entire model, tailored specifically to the subject, thereby enabling better personalization.
In this post, you will explore the following concepts:
- Fine-tuning challenges and recommended settings in DreamBooth
- Stable Diffusion fine-tuning with DreamBooth –