TOOD: Task-aligned One-stage Object Detection
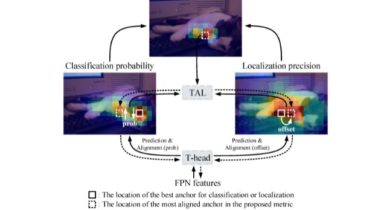
TOOD
TOOD: Task-aligned One-stage Object Detection (ICCV 2021 Oral)
One-stage object detection is commonly implemented by optimizing two sub-tasks: object classification and localization, using heads with two parallel branches, which might lead to a certain level of spatial misalignment in predictions between the two tasks. In this work, we propose a Task-aligned One-stage Object Detection (TOOD) that explicitly aligns the two tasks in a learning-based manner. First, we design a novel Task-aligned Head (T-Head) which offers a better balance between learning task-interactive and task-specific features, as well as a greater flexibility to learn the alignment via a task-aligned predictor. Second, we propose Task Alignment Learning (TAL) to explicitly pull closer (or even unify) the optimal anchors for the two tasks during training via a designed sample assignment scheme and