The Gap on GAP: Tackling the Problem of Differing Data Distributions in Bias-Measuring Datasets
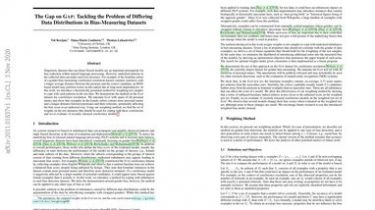
Diagnostic datasets that can detect biased models are an important prerequisite for bias reduction within natural language processing. However, undesired patterns in the collected data can make such tests incorrect...
For example, if the feminine subset of a gender-bias-measuring coreference resolution dataset contains sentences with a longer average distance between the pronoun and the correct candidate, an RNN-based model may perform worse on this subset due to long-term dependencies. In this work, we introduce a theoretically grounded method for weighting test samples to cope with such patterns in the test data. We demonstrate the method on