Suppressing Mislabeled Data via Grouping and Self-Attention
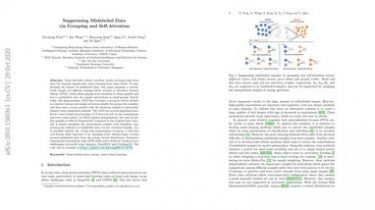
Deep networks achieve excellent results on large-scale clean data but degrade significantly when learning from noisy labels. To suppressing the impact of mislabeled data, this paper proposes a conceptually simple yet efficient training block, termed as Attentive Feature Mixup (AFM), which allows paying more attention to clean samples and less to mislabeled ones via sample interactions in small groups...
Specifically, this plug-and-play AFM first leverages a textit{group-to-attend} module to construct groups and assign attention weights for group-wise samples, and then uses a textit{mixup} module with the attention weights to interpolate massive noisy-suppressed samples. The AFM has