SRF-GAN: Super-Resolved Feature GAN for Multi-Scale Representation
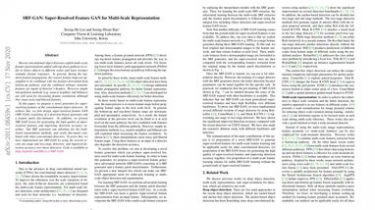
Recent convolutional object detectors exploit multi-scale feature representations added with top-down pathway in order to detect objects at different scales and learn stronger semantic feature responses. In general, during the top-down feature propagation, the coarser feature maps are upsampled to be combined with the features forwarded from bottom-up pathway, and the combined stronger semantic features are inputs of detector’s headers...
However, simple interpolation methods (e.g. nearest neighbor and bilinear) are still used for increasing feature resolutions although they cause noisy and blurred features. In this paper, we propose a novel generator for super-resolving features of the