SnapMix: Semantically Proportional Mixing for Augmenting Fine-grained Data
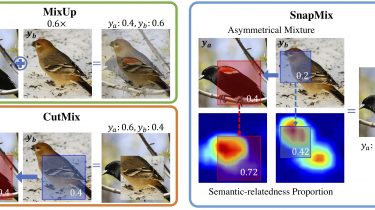
Data mixing augmentation has proved effective in training deep models. Recent methods mix labels mainly based on the mixture proportion of image pixels...
As the main discriminative information of a fine-grained image usually resides in subtle regions, methods along this line are prone to heavy label noise in fine-grained recognition. We propose in this paper a novel scheme, termed as Semantically Proportional Mixing (SnapMix), which exploits class activation map (CAM) to lessen the label noise in augmenting fine-grained data. SnapMix generates the target label for a mixed image by estimating its intrinsic semantic composition, and allows