Simple statistical methods for unsupervised brain anomaly detection on MRI are competitive to deep learning methods
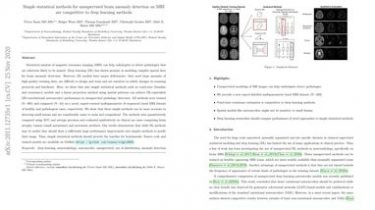
Statistical analysis of magnetic resonance imaging (MRI) can help radiologists to detect pathologies that are otherwise likely to be missed. Deep learning (DL) has shown promise in modeling complex spatial data for brain anomaly detection...
However, DL models have major deficiencies: they need large amounts of high-quality training data, are difficult to design and train and are sensitive to subtle changes in scanning protocols and hardware. Here, we show that also simple statistical methods such as voxel-wise (baseline and covariance) models and a linear projection method using spatial patterns can achieve DL-equivalent (3D convolutional autoencoder) performance