Semi-Supervised Learning With Label Spreading
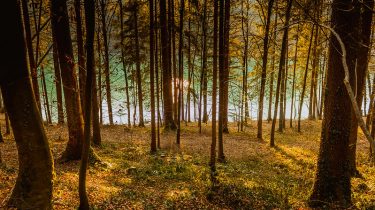
Semi-supervised learning refers to algorithms that attempt to make use of both labeled and unlabeled training data.
Semi-supervised learning algorithms are unlike supervised learning algorithms that are only able to learn from labeled training data.
A popular approach to semi-supervised learning is to create a graph that connects examples in the training dataset and propagates known labels through the edges of the graph to label unlabeled examples. An example of this approach to semi-supervised learning is the label spreading algorithm for classification predictive modeling.
In this tutorial, you will discover how to apply the label spreading algorithm to a semi-supervised learning classification dataset.
After completing this tutorial, you will know:
- An intuition for how the label spreading semi-supervised learning