Rethinking Space-Time Networks with Improved Memory Coverage for Efficient Video Object Segmentation
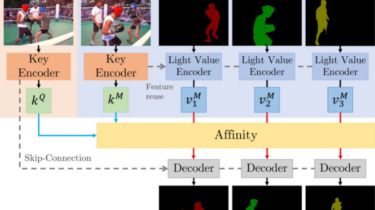
STCN
We present Space-Time Correspondence Networks (STCN) as the new, effective, and efficient framework to model space-time correspondences in the context of video object segmentation. STCN achieves SOTA results on multiple benchmarks while running fast at 20+ FPS without bells and whistles. Its speed is even higher with mixed precision. Despite its effectiveness, the network itself is very simple with lots of room for improvement. See the paper for technical details.
A Gentle Introduction
There are two main contributions: STCN framework (above figure), and L2 similarity. We build affinity between images instead of between (image, mask) pairs — this leads to a significantly speed up, memory saving (because we compute one, instead of multiple affinity matrices), and robustness. We