Rethinking Learnable Tree Filter for Generic Feature Transform
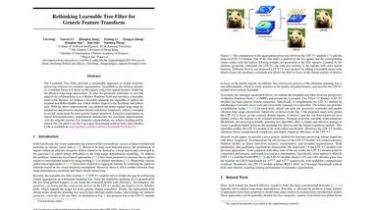
The Learnable Tree Filter presents a remarkable approach to model structure-preserving relations for semantic segmentation. Nevertheless, the intrinsic geometric constraint forces it to focus on the regions with close spatial distance, hindering the effective long-range interactions...
To relax the geometric constraint, we give the analysis by reformulating it as a Markov Random Field and introduce a learnable unary term. Besides, we propose a learnable spanning tree algorithm to replace the original non-differentiable one, which further improves the flexibility and robustness. With the above improvements, our method can better capture long range dependencies and preserve structural details