Rel3D: A Minimally Contrastive Benchmark for Grounding Spatial Relations in 3D
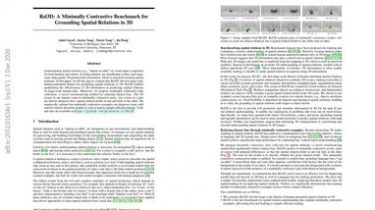
Understanding spatial relations (e.g., “laptop on table”) in visual input is important for both humans and robots. Existing datasets are insufficient as they lack large-scale, high-quality 3D ground truth information, which is critical for learning spatial relations...
In this paper, we fill this gap by constructing Rel3D: the first large-scale, human-annotated dataset for grounding spatial relations in 3D. Rel3D enables quantifying the effectiveness of 3D information in predicting spatial relations on large-scale human data. Moreover, we propose minimally contrastive data collection — a novel crowdsourcing method for reducing dataset bias. The 3D scenes in our dataset