Reconstructing cellular automata rules from observations at nonconsecutive times
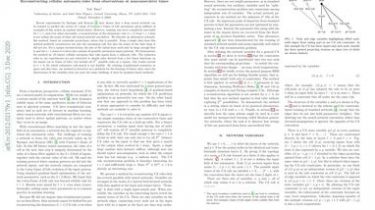
Recent experiments by Springer and Kenyon have shown that a deep neural network can be trained to predict the action of $t$ steps of Conway’s Game of Life automaton given millions of examples of this action on random initial states. However, training was never completely successful for $t>1$, and even when successful, a reconstruction of the elementary rule ($t=1$) from $t>1$ data is not within the scope of what the neural network can deliver...
We describe an alternative network-like method, based on constraint projections, where this is possible. From a single data item this method perfectly