Pytorch Code and Data for EnvEdit: Environment Editing for Vision-and-Language Navigation (CVPR 2022)
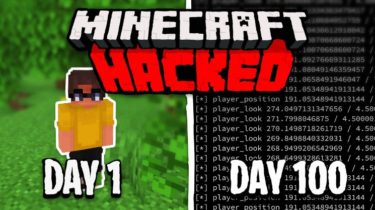
In Vision-and-Language Navigation (VLN), an agent needs to navigate through the environment based on natural language instructions.
Due to limited available data for agent training and finite diversity in navigation environments, it is challenging for the agent to generalize to new, unseen environments.
To address this problem, we propose EnvEdit, a data augmentation method that creates new environments by editing existing environments, which are used to train a more generalizable agent.
Our augmented environments can differ from the seen environments in three diverse aspects: style, object appearance, and object classes.
Training on these edit-augmented environments prevents the agent from overfitting to existing environments and helps generalize better to new, unseen environments.
Empirically, on both the Room-to-Room and the multi-lingual Room-Across-Room datasets, we show that our proposed EnvEdit method gets