Pixel-level self-paced learning for super-resolution
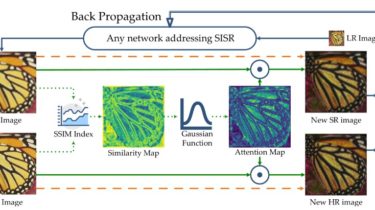
This is an official implementaion of the paper Pixel-level Self-Paced Learning for Super-Resolution, which has been accepted by ICASSP 2020.
trained model files: Baidu Pan(code: v0be)
Requirements
This code is forked from thstkdgus35/EDSR-PyTorch. In the light of its README, following libraries are required:
- Python 3.6+ (Python 3.7.0 in my experiments)
- PyTorch >= 1.0.0 (1.1.0 in my experiments)
- numpy
- skimage
- imageio
- matplotlib
- tqdm
Core Parts
Detail code can be found in Loss.forward, which can be simplified as:
# take L1 Loss as example
import torch
import torch.nn as nn
import torch.nn.functional as F
from . import pytorch_ssim
class Loss(nn.modules.loss._Loss):
def __init__(self, spl_alpha, spl_beta, spl_maxVal):
super(Loss, self).__init__()
self.loss = nn.L1Loss()
self.alpha = spl_alpha
self.beta = spl_beta
self.maxVal = spl_maxVal