Multivariate Time Series Forecasting with LSTMs in Keras
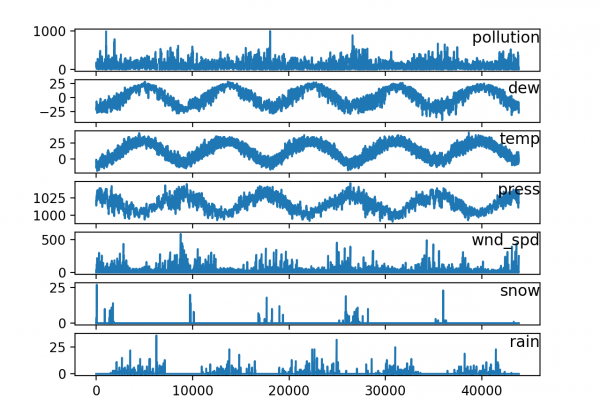
Last Updated on August 28, 2020
Neural networks like Long Short-Term Memory (LSTM) recurrent neural networks are able to almost seamlessly model problems with multiple input variables.
This is a great benefit in time series forecasting, where classical linear methods can be difficult to adapt to multivariate or multiple input forecasting problems.
In this tutorial, you will discover how you can develop an LSTM model for multivariate time series forecasting with the Keras deep learning library.
After completing this tutorial, you will know:
- How to transform a raw dataset into something we can use for time series forecasting.
- How to prepare data and fit an LSTM for a multivariate time series forecasting problem.
- How to make a forecast and rescale the result back into the original units.
Kick-start your project with my new book Deep Learning for Time Series Forecasting, including step-by-step tutorials and the Python source code files for all examples.
Let’s get started.
- Update Aug/2017: Fixed a bug where yhat was compared to obs at the previous time step when calculating the final RMSE. Thanks, Songbin Xu and David Righart.
- Update Oct/2017: Added a new example showing how to train on multiple prior time steps
To finish reading, please visit source site