ML and NLP Research Highlights of 2020
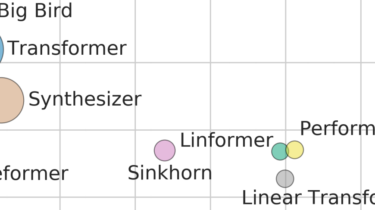
The selection of areas and methods is heavily influenced by my own interests; the selected topics are biased towards representation and transfer learning and towards natural language processing (NLP). I tried to cover the papers that I was aware of but likely missed many relevant ones—feel free to highlight them in the comments below. In all, I discuss the following highlights:
- Scaling up—and down
- Retrieval augmentation
- Few-shot learning
- Contrastive learning
- Evaluation beyond accuracy
- Practical concerns of large LMs
- Multilinguality
- Image Transformers
- ML for science
- Reinforcement learning
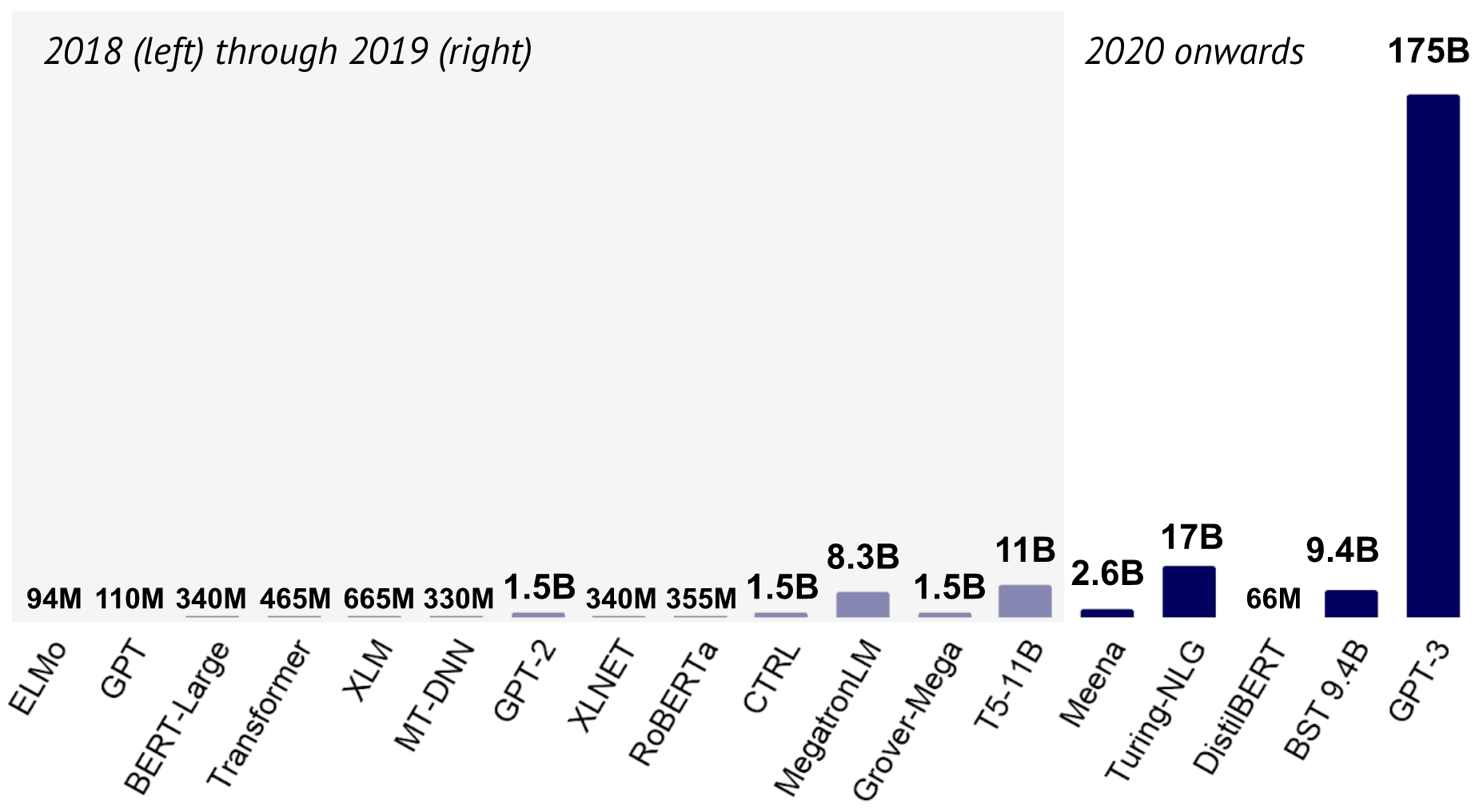
What