Many-Speakers Single Channel Speech Separation with Optimal Permutation Training
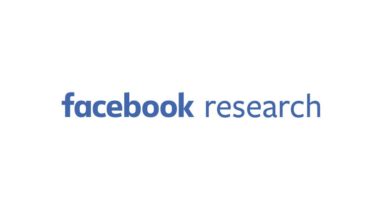
Abstract
Single channel speech separation has experienced great progress in the last few years. However, training neural speech separation for a large number of speakers (e.g., more than 10 speakers) is out of reach for the current methods, which rely on the Permutation Invariant Training (PIT). In this work, we present a permutation invariant training that employs the Hungarian algorithm in order to train with an O (C 3) time complexity, where C is the number of speakers, in comparison to O(C !) of PIT based methods. Furthermore, we present a modified architecture that can handle the increased number