Machine Learning – Anomaly Detection: “Finding a Needle in a Haystack”
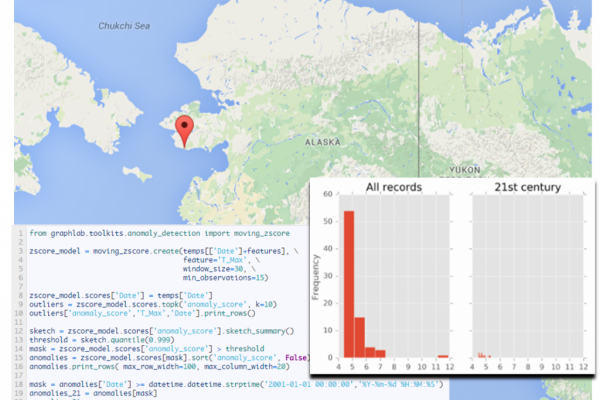
After exploring formulation, classification, benchmarking, we explore another facet of Machine Learning: anomaly detection. This part is key in the IoT transformation, as it enables internet-connected AI devices to alert, adapt and respond accordingly. Once properly trained, an IoT could not only warn and prevent imminent failure, but also execute a response, adaptive to the anomaly detected.
In this process, we’ll explore intrinsic hurdles that makes the anomaly detection process a non-trivial task of “finding a needle in haystack”.
Opportunities abound to explore, and any univariate sequential dataset (time series) can be used to demonstrate applicability. For this illustrative purpose, a common public source is certainly weather data.
Medias have been covering extensively ‘global warming’ and climate changes at the planetary level. Recently, the COP21 saw many warming trends exposed and targets projected. In view of current observations in the northern hemisphere, we choose to analyze specific data trends to see if anomalies could be detected, and understand the complexity of this process. We also explore FFT filtering that can be applied to periodic signals, and compare original and filtered signal