Low-Complexity Models for Acoustic Scene Classification Based on Receptive Field Regularization and Frequency Damping
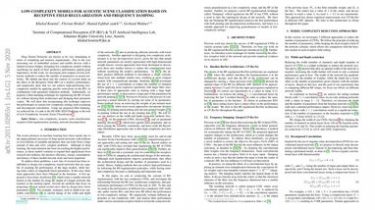
Deep Neural Networks are known to be very demanding in terms of computing and memory requirements. Due to the ever increasing use of embedded systems and mobile devices with a limited resource budget, designing low-complexity models without sacrificing too much of their predictive performance gained great importance...
In this work, we investigate and compare several well-known methods to reduce the number of parameters in neural networks. We further put these into the context of a recent study on the effect of the Receptive Field (RF) on a model’s performance, and empirically show that we can achieve