Learning to Use Future Information in Simultaneous Translation
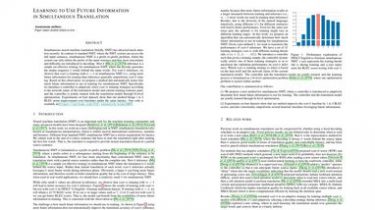
Simultaneous neural machine translation (briefly, NMT) has attracted much attention recently. In contrast to standard NMT, where the NMT system can access the full input sentence, simultaneous NMT is a prefix-to-prefix problem, where the system can only utilize the prefix of the input sentence and thus more uncertainty and difficulty are introduced to decoding...
Wait-k inference is a simple yet effective strategy for simultaneous NMT, where the decoder generates the output sequence $k$ words behind the input words. For wait-k inference, we observe that wait-m training with $m>k$ in simultaneous NMT (i.e., using more future information