Learning Deep Interleaved Networks with Asymmetric Co-Attention for Image Restoration
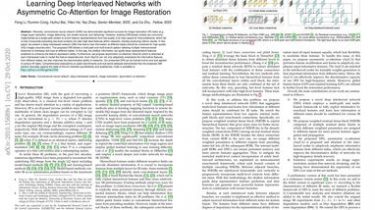
Recently, convolutional neural network (CNN) has demonstrated significant success for image restoration (IR) tasks (e.g., image super-resolution, image deblurring, rain streak removal, and dehazing). However, existing CNN based models are commonly implemented as a single-path stream to enrich feature representations from low-quality (LQ) input space for final predictions, which fail to fully incorporate preceding low-level contexts into later high-level features within networks, thereby producing inferior results...
In this paper, we present a deep interleaved network (DIN) that learns how information at different states should be combined for high-quality (HQ) images reconstruction. The proposed DIN follows a