Information-Theoretic Visual Explanation for Black-Box Classifiers
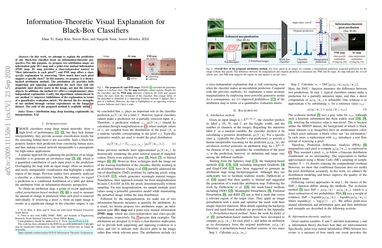
In this work, we attempt to explain the prediction of any black-box classifier from an information-theoretic perspective. For this purpose, we propose two attribution maps: an information gain (IG) map and a point-wise mutual information (PMI) map...
IG map provides a class-independent answer to “How informative is each pixel? “, and PMI map offers a class-specific explanation by answering “How much does each pixel support a specific class?” In this manner, we propose (i) a theory-backed attribution method. The attribution (ii) provides both supporting and opposing explanations for each class and (iii) pinpoints most decisive parts in the image, not just the relevant objects. In addition, the method (iv) offers a complementary class-independent explanation. Lastly, the algorithmic enhancement in our method (v) improves faithfulness of the explanation in terms of a quantitative evaluation metric. We showed the five strengths of our method through various experiments on the ImageNet dataset. The code of the proposed method is available online.