How to Tune ARIMA Parameters in Python
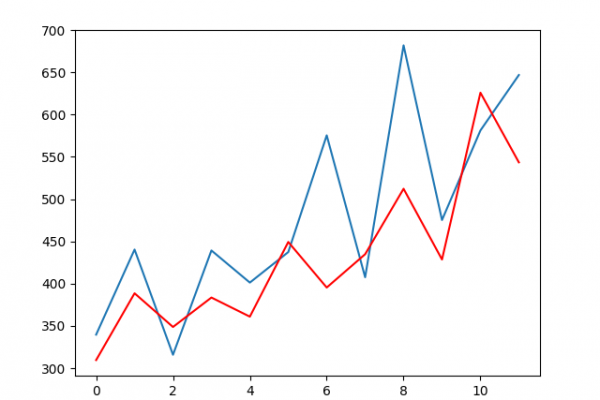
Last Updated on August 21, 2019
There are many parameters to consider when configuring an ARIMA model with Statsmodels in Python.
In this tutorial, we take a look at a few key parameters (other than the order parameter) that you may be curious about.
Specifically, after completing this tutorial, you will know:
- How to suppress noisy output from the underlying mathematical libraries when fitting an ARIMA model.
- The effect of enabling or disabling a trend term in your ARIMA model.
- The influence of using different mathematical solvers to fit coefficients to your training data.
Note, if you are interested in tuning the order parameter, see the post:
Kick-start your project with my new book Time Series Forecasting With Python, including step-by-step tutorials and the Python source code files for all examples.
Let’s get started.
- Updated Apr/2019: Updated the link to dataset.
Shampoo Sales Dataset
This dataset describes the monthly number of sales of shampoo over a 3 year period.
The units are a sales count and there are 36 observations. The original dataset is credited to Makridakis, Wheelwright, and Hyndman (1998).
The example below loads and creates a plot of the loaded dataset.