How to Transform Data to Better Fit The Normal Distribution
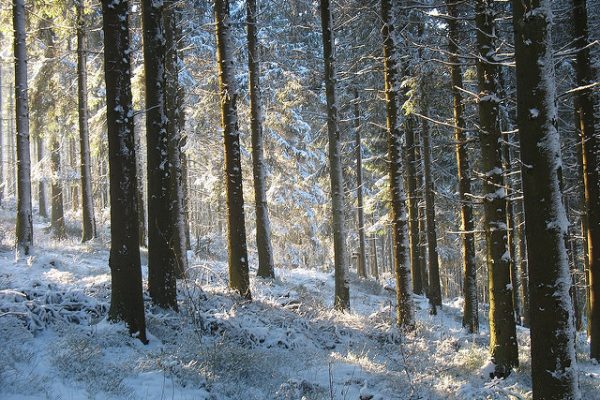
Last Updated on August 8, 2019
A large portion of the field of statistics is concerned with methods that assume a Gaussian distribution: the familiar bell curve.
If your data has a Gaussian distribution, the parametric methods are powerful and well understood. This gives some incentive to use them if possible. Even if your data does not have a Gaussian distribution.
It is possible that your data does not look Gaussian or fails a normality test, but can be transformed to make it fit a Gaussian distribution. This is more likely if you are familiar with the process that generated the observations and you believe it to be a Gaussian process, or the distribution looks almost Gaussian, except for some distortion.
In this tutorial, you will discover the reasons why a Gaussian-like distribution may be distorted and techniques that you can use to make a data sample more normal.
After completing this tutorial, you will know:
- How to consider the size of the sample and whether the law of large numbers may help improve the distribution of a sample.
- How to identify and remove extreme values and long tails from a distribution.
- Power transforms and the Box-Cox transform
To finish reading, please visit source site