How to Scale Data for Long Short-Term Memory Networks in Python
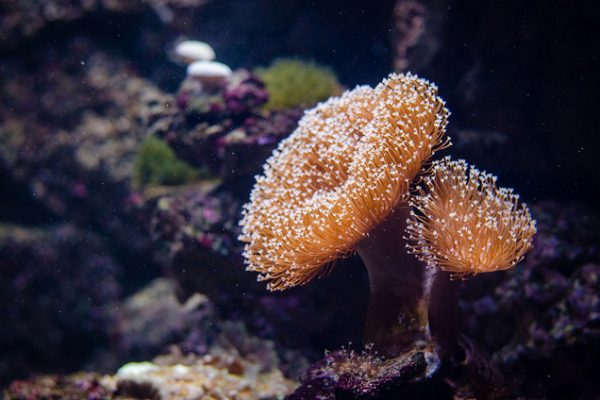
Last Updated on August 5, 2019
The data for your sequence prediction problem probably needs to be scaled when training a neural network, such as a Long Short-Term Memory recurrent neural network.
When a network is fit on unscaled data that has a range of values (e.g. quantities in the 10s to 100s) it is possible for large inputs to slow down the learning and convergence of your network and in some cases prevent the network from effectively learning your problem.
In this tutorial, you will discover how to normalize and standardize your sequence prediction data and how to decide which to use for your input and output variables.
After completing this tutorial, you will know:
- How to normalize and standardize sequence data in Python.
- How to select the appropriate scaling for input and output variables.
- Practical considerations when scaling sequence data.
Kick-start your project with my new book Deep Learning for Time Series Forecasting, including step-by-step tutorials and the Python source code files for all examples.
Let’s get started.
