How to Model Volatility with ARCH and GARCH for Time Series Forecasting in Python
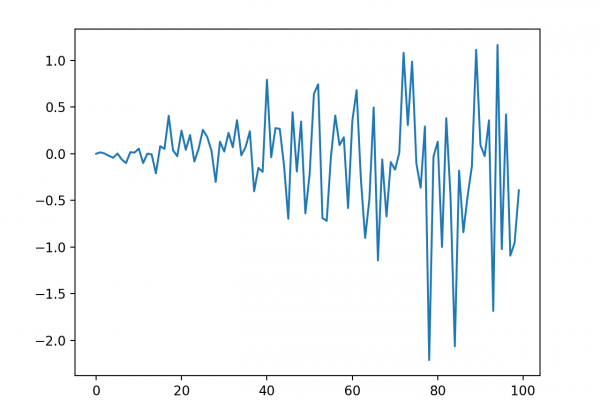
Last Updated on August 21, 2019
A change in the variance or volatility over time can cause problems when modeling time series with classical methods like ARIMA.
The ARCH or Autoregressive Conditional Heteroskedasticity method provides a way to model a change in variance in a time series that is time dependent, such as increasing or decreasing volatility. An extension of this approach named GARCH or Generalized Autoregressive Conditional Heteroskedasticity allows the method to support changes in the time dependent volatility, such as increasing and decreasing volatility in the same series.
In this tutorial, you will discover the ARCH and GARCH models for predicting the variance of a time series.
After completing this tutorial, you will know:
- The problem with variance in a time series and the need for ARCH and GARCH models.
- How to configure ARCH and GARCH models.
- How to implement ARCH and GARCH models in Python.
Kick-start your project with my new book Time Series Forecasting With Python, including step-by-step tutorials and the Python source code files for all examples.
Let’s get started.
