Highlights from Machine Translation and Multilinguality in December 2024 and January 2025
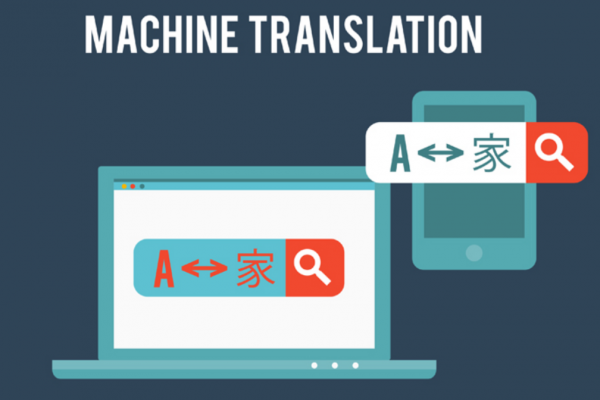
MuLan: Adapting Multilingual Diffusion Models for Hundreds of Languages with Negligible Cost
Researchers from Tsinghua, Shanghai, Beijing, Hong Kong, and Johns Hopkins have developed a method for adapting diffusion models to hundreds of languages at a minimal cost. They achieve this by swapping the text encoder with a multilingual one and training it to produce representations consistent with the CLIP encoder, leveraging parallel language data and English image-text data. The results look impressive and multilingual, and the generation quality, as measured by CLIP representation similarity, appears promising (although I am not really sure how convincing automatic evaluation can be in such cases).
On The Origin of Cultural Biases in Language Models: From Pre-training Data to Linguistic Phenomena
Folks from Georgia Tech observe that LLMs in Arabic