FixBi: Bridging Domain Spaces for Unsupervised Domain Adaptation
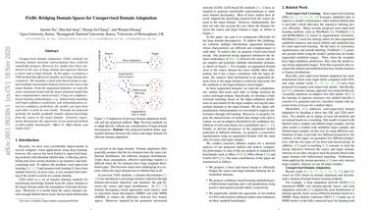
Unsupervised domain adaptation (UDA) methods for learning domain invariant representations have achieved remarkable progress. However, few studies have been conducted on the case of large domain discrepancies between a source and a target domain...
In this paper, we propose a UDA method that effectively handles such large domain discrepancies. We introduce a fixed ratio-based mixup to augment multiple intermediate domains between the source and target domain. From the augmented-domains, we train the source-dominant model and the target-dominant model that have complementary characteristics. Using our confidence-based learning methodologies, e.g., bidirectional matching with high-confidence predictions and self-penalization using