Fast, differentiable sorting and ranking in PyTorch
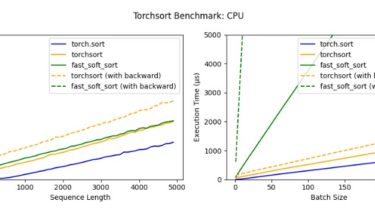
Torchsort
Pure PyTorch implementation of Fast Differentiable Sorting and Ranking (Blondel et al.). Much of the code is copied from the original Numpy implementation at google-research/fast-soft-sort, with the isotonic regression solver rewritten as a PyTorch C++ and CUDA extension.
Install
pip install torchsort
To build the CUDA extension you will need the CUDA toolchain installed. If you
want to build in an environment without a CUDA runtime (e.g. docker), you will
need to export the environment variableTORCH_CUDA_ARCH_LIST="Pascal;Volta;Turing"
before installing.
Usage
torchsort
exposes two functions: soft_rank
and soft_sort
, each with
parameters regularization
("l2"
or "kl"
) and regularization_strength
(a
scalar value). Each will rank/sort the last dimension of a 2-d tensor, with an
accuracy dependant upon the regularization strength:
import torch
import torchsort
x = torch.tensor([[8, 0, 5, 3, 2, 1,