Evaluating the Factual Consistency of Abstractive Text Summarization
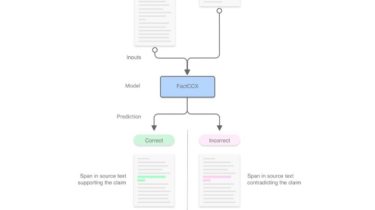
factCC
Evaluating the Factual Consistency of Abstractive Text Summarization
Authors: Wojciech Kryściński, Bryan McCann, Caiming Xiong, and Richard Socher
Introduction
Currently used metrics for assessing summarization algorithms do not account for whether summaries are factually consistent with source documents.
We propose a weakly-supervised, model-based approach for verifying factual consistency and identifying conflicts between source documents and a generated summary.
Training data is generated by applying a series of rule-based transformations to the sentences of source documents.
The factual consistency model is then trained jointly for three tasks:
- identify whether sentences remain factually consistent after transformation,
- extract a span in the source documents to support the consistency prediction,
- extract a span in the summary sentence that is inconsistent if one exists.
Transferring this model to summaries generated by several state-of-the art models reveals that