Error-Correcting Output Codes (ECOC) for Machine Learning
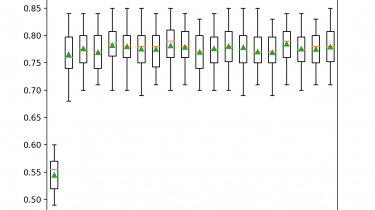
Machine learning algorithms, like logistic regression and support vector machines, are designed for two-class (binary) classification problems.
As such, these algorithms must either be modified for multi-class (more than two) classification problems or not used at all. The Error-Correcting Output Codes method is a technique that allows a multi-class classification problem to be reframed as multiple binary classification problems, allowing the use of native binary classification models to be used directly.
Unlike one-vs-rest and one-vs-one methods that offer a similar solution by dividing a multi-class classification problem into a fixed number of binary classification problems, the error-correcting output codes technique allows each class to be encoded as an arbitrary number of binary classification problems. When an overdetermined representation