Enhancing Diversity in Teacher-Student Networks via Asymmetric branches for Unsupervised Person Re-identification
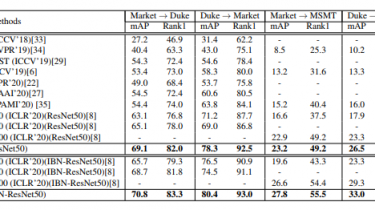
The objective of unsupervised person re-identification (Re-ID) is to learn discriminative features without labor-intensive identity annotations. State-of-the-art unsupervised Re-ID methods assign pseudo labels to unlabeled images in the target domain and learn from these noisy pseudo labels...
Recently introduced Mean Teacher Model is a promising way to mitigate the label noise. However, during the training, self-ensembled teacher-student networks quickly converge to a consensus which leads to a local minimum. We explore the possibility of using an asymmetric structure inside neural network to address this problem. First, asymmetric branches are proposed to extract features in different manners,