Efficient semidefinite-programming-based inference for binary and multi-class MRFs
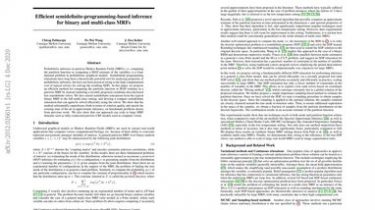
Probabilistic inference in pairwise Markov Random Fields (MRFs), i.e. computing the partition function or computing a MAP estimate of the variables, is a foundational problem in probabilistic graphical models. Semidefinite programming relaxations have long been a theoretically powerful tool for analyzing properties of probabilistic inference, but have not been practical owing to the high computational cost of typical solvers for solving the resulting SDPs...
In this paper, we propose an efficient method for computing the partition function or MAP estimate in a pairwise MRF by instead exploiting a recently proposed coordinate-descent-based fast semidefinite solver. We also