Efficient Deep Neural Network Training via Cyclic Precision
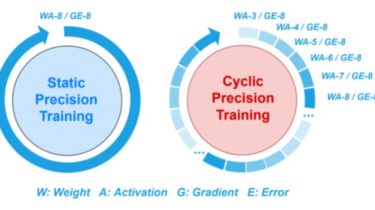
CPT
Yonggan Fu, Han Guo, Meng Li, Xin Yang, Yining Ding, Vikas Chandra, Yingyan Lin
Accepted at ICLR 2021 (Spotlight) [Paper Link].
Overview
Low-precision deep neural network (DNN) training has gained tremendous attention as reducing precision is one of the most effective knobs for boosting DNNs’ training time/energy efficiency. In this paper, we attempt to explore low-precision training from a new perspective as inspired by recent findings in understanding DNN training: we conjecture that DNNs’ precision might have a similar effect as the learning rate during DNN training, and advocate dynamic precision along the training trajectory for further boosting the time/energy efficiency of DNN training. Specifically, we propose Cyclic Precision Training (CPT) to cyclically vary the precision between two boundary values to balance the coarse-grained exploration of