Domain adaptation under structural causal models
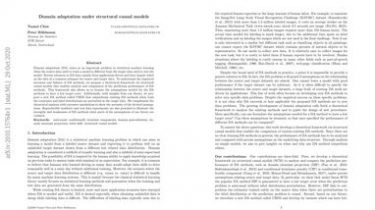
Domain adaptation (DA) arises as an important problem in statistical machine learning when the source data used to train a model is different from the target data used to test the model. Recent advances in DA have mainly been application-driven and have largely relied on the idea of a common subspace for source and target data...
To understand the empirical successes and failures of DA methods, we propose a theoretical framework via structural causal models that enables analysis and comparison of the prediction performance of DA methods. This framework also allows us to itemize the assumptions