DoLFIn: Distributions over Latent Features for Interpretability
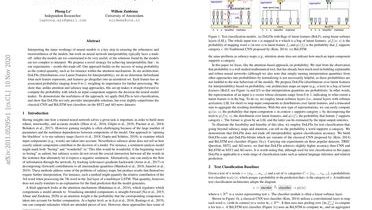
Interpreting the inner workings of neural models is a key step in ensuring the robustness and trustworthiness of the models, but work on neural network interpretability typically faces a trade-off: either the models are too constrained to be very useful, or the solutions found by the models are too complex to interpret. We propose a novel strategy for achieving interpretability that — in our experiments — avoids this trade-off...
Our approach builds on the success of using probability as the central quantity, such as for instance within the attention mechanism. In our architecture, DoLFIn (Distributions over