Comparing Classical and Machine Learning Algorithms for Time Series Forecasting
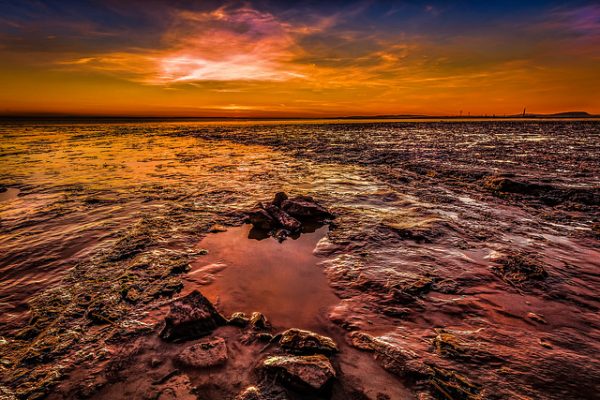
Last Updated on August 5, 2019
Machine learning and deep learning methods are often reported to be the key solution to all predictive modeling problems.
An important recent study evaluated and compared the performance of many classical and modern machine learning and deep learning methods on a large and diverse set of more than 1,000 univariate time series forecasting problems.
The results of this study suggest that simple classical methods, such as linear methods and exponential smoothing, outperform complex and sophisticated methods, such as decision trees, Multilayer Perceptrons (MLP), and Long Short-Term Memory (LSTM) network models.
These findings highlight the requirement to both evaluate classical methods and use their results as a baseline when evaluating any machine learning and deep learning methods for time series forecasting in order demonstrate that their added complexity is adding skill to the forecast.
In this post, you will discover the important findings of this recent study evaluating and comparing the performance of a classical and modern machine learning methods on a large and diverse set of time series forecasting datasets.
After reading this post, you will know:
- Classical methods like ETS and ARIMA out-perform machine learning and deep learning methods
To finish reading, please visit source site