CatBoost Essentials: Building Robust Home Price Prediction Systems
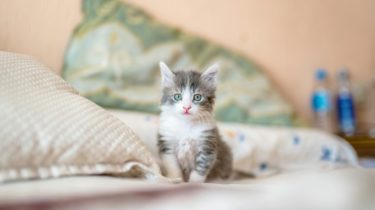
Gradient boosting algorithms are powerful tools for prediction tasks, and CatBoost has gained popularity for its efficient handling of categorical data. This is especially valuable for the Ames Housing dataset, which contains numerous categorical features such as neighborhood, house style, and sale condition.
CatBoost excels with categorical features through its innovative “ordered target statistics” approach. Unlike traditional methods that require extensive preprocessing (like one-hot encoding), CatBoost can work directly with categorical variables. It calculates statistics on the target variable for each category, considering the ordering of examples to prevent overfitting.
In this post, we will explore CatBoost’s unique features, such as Symmetric Trees and Ordered Boosting, and compare different configurations. You’ll learn how to implement CatBoost for regression, prepare