Best practices of orchestrating Python and R code in ML projects
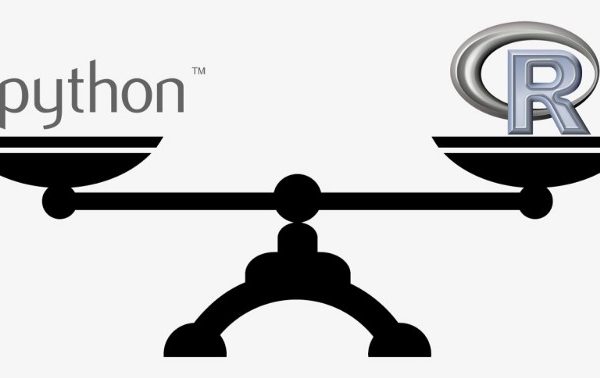
Today, data scientists are generally divided among two languages — some prefer R, some prefer Python. I will not try to explain in this article which one is better. Instead of that I will try to find an answer to a question: “What is the best way to integrate both languages in one data science project? What are the best practices?”. Beside git and shell scripting additional tools are developed to facilitate the development of predictive model in a multi-language environments. For fast data exchange between R and Python let’s use binary data file format Feather. Another language agnostic tool DVC can make the research reproducible — let’s use DVC to orchestrate R and Python code instead of a regular shell scripts.
Machine learning with R and Python
Both R and Python are having powerful libraries/packages used for predictive modeling. Usually algorithms used for classification or regression are implemented in both languages and some scientist are using R while some of them preferring Python. we use a target variable with binary output and logistic regression was used as a training algorithm.