A Lightweight and Stable DRL Implementation Using PyTorch
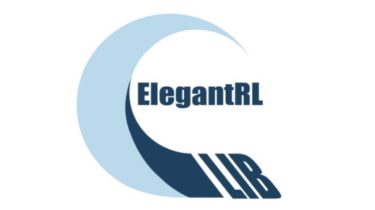
ElegantRL
ElegantRL is featured with lightweight, efficient and stable, for researchers and practitioners.
- Lightweight: The core codes <1,000 lines (check elegantrl/tutorial), using PyTorch (train), OpenAI Gym (env), NumPy, Matplotlib (plot).
- Efficient: performance is comparable with Ray RLlib.
- Stable: as stable as Stable Baseline 3.
Currently, model-free deep reinforcement learning (DRL) algorithms:
- DDPG, TD3, SAC, A2C, PPO, PPO(GAE) for continuous actions
- DQN, DoubleDQN, D3QN for discrete actions
For DRL algorithms, please check out the educational webpage OpenAI Spinning Up.
File Structure
An agent in agent.py uses networks in net.py and is trained in run.py by interacting with an environment in env.py.
—–kernel file—-
- elegantrl/net.py # Neural networks.
- Q-Net,
- Actor Network,
- Critic Network,
- elegantrl/agent.py # RL algorithms.
- elegantrl/run.py # run DEMO 1