A Gradient Flow Framework For Analyzing Network Pruning
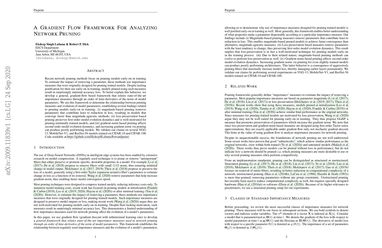
Recent network pruning methods focus on pruning models early-on in training. To estimate the impact of removing a parameter, these methods use importance measures that were originally designed for pruning trained models...
Despite lacking justification for their use early-on in training, models pruned using such measures result in surprisingly minimal accuracy loss. To better explain this behavior, we develop a general, gradient-flow based framework that relates state-of-the-art importance measures through an order of time-derivative of the norm of model parameters. We use this framework to determine the relationship between pruning measures and evolution of model parameters, establishing several findings related to pruning models early-on in training: (i) magnitude-based pruning removes parameters that contribute least to reduction in loss, resulting in models that converge faster than magnitude-agnostic methods; (ii) loss-preservation based pruning preserves first-order model evolution dynamics and is well-motivated for pruning minimally trained models; and (iii) gradient-norm based pruning affects second-order model evolution dynamics, and increasing gradient norm via pruning can produce poorly performing models. We validate our claims on several VGG-13, MobileNet-V1, and ResNet-56 models trained on CIFAR-10 and CIFAR-100. Code available at https://github.com/EkdeepSLubana/flowandprune.