A Gentle Introduction to StyleGAN the Style Generative Adversarial Network
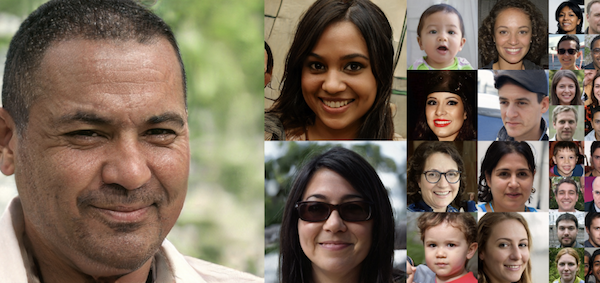
Last Updated on May 10, 2020
Generative Adversarial Networks, or GANs for short, are effective at generating large high-quality images.
Most improvement has been made to discriminator models in an effort to train more effective generator models, although less effort has been put into improving the generator models.
The Style Generative Adversarial Network, or StyleGAN for short, is an extension to the GAN architecture that proposes large changes to the generator model, including the use of a mapping network to map points in latent space to an intermediate latent space, the use of the intermediate latent space to control style at each point in the generator model, and the introduction to noise as a source of variation at each point in the generator model.
The resulting model is capable not only of generating impressively photorealistic high-quality photos of faces, but also offers control over the style of the generated image at different levels of detail through varying the style vectors and noise.
In this post, you will discover the Style Generative Adversarial Network that gives control over the style of generated synthetic images.
After reading this post, you will know:
- The lack of control over the style of
To finish reading, please visit source site