Gradient Descent Optimization With AMSGrad From Scratch
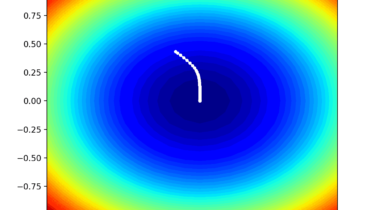
Gradient descent is an optimization algorithm that follows the negative gradient of an objective function in order to locate the minimum of the function.
A limitation of gradient descent is that a single step size (learning rate) is used for all input variables. Extensions to gradient descent like the Adaptive Movement Estimation (Adam) algorithm use a separate step size for each input variable but may result in a step size that rapidly decreases to very small values.
AMSGrad is an extension to the Adam version of gradient descent that attempts to improve the convergence properties of the algorithm, avoiding large abrupt changes in the learning rate for each input variable.
In this tutorial, you will discover how to