Machine Learning that Matters
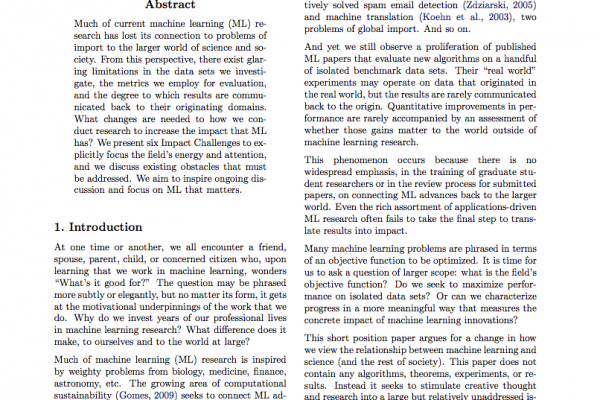
Last Updated on September 5, 2016
Reading bootstrapping machine learning, Louis mentioned a paper that I had to go off and read. The title of the paper is Machine Learning that Matters (PDF) by Kiri Wagstaff from JPL and was published in 2012.
Kiri’s thesis is that the machine learning research community has lost its way. She suggests that much of machine learning is done for machine learning’s sake. She points to three key problems:
- Overfocus on Benchmark Data: Focus on datasets in UCI repository, but very few make an impact in the domain that is being addressed. She points to the lack of standards for experiment reproducability which void the use of standard datasets and the skew towards regression and classification problems. She comments that using the UCI repository is worse than using synthetic daa because we don’t even have control over how the data was created.
- Overfocus on Abstract Metrics: A strong focus on algorithm racing or bake-offs and the use of
To finish reading, please visit source site