Model Prediction Accuracy Versus Interpretation in Machine Learning
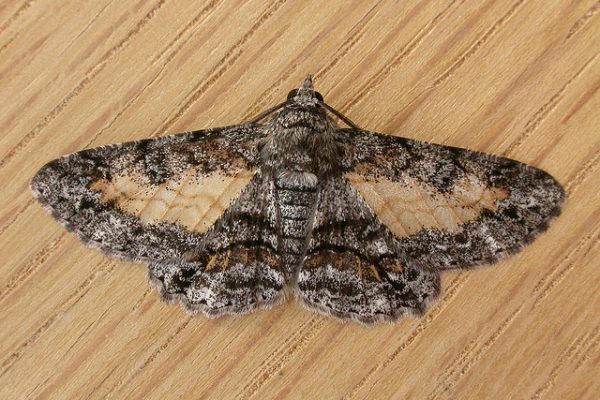
Last Updated on August 15, 2020
In their book Applied Predictive Modeling, Kuhn and Johnson comment early on the trade-off of model prediction accuracy versus model interpretation.
For a given problem, it is critical to have a clear idea of the which is a priority, accuracy or explainability so that this trade-off can be made explicitly rather than implicitly.
In this post you will discover and consider this important trade-off.
Accuracy and Explainability
Model performance is estimated in terms of its accuracy to predict the occurrence of an event on unseen data. A more accurate model is seen as a more valuable model.
Model interpretability provides insight into the relationship between in the inputs and the output. An interpreted model can answer questions as to why the independent features predict the dependent attribute.
The issue arises because as model accuracy increases so does model complexity, at the cost of interpretability.
Model Complexity
A model with higher the accuracy can mean more opportunities, benefits,
To finish reading, please visit source site