Highlights from Machine Translation and Multilinguality in October 2022
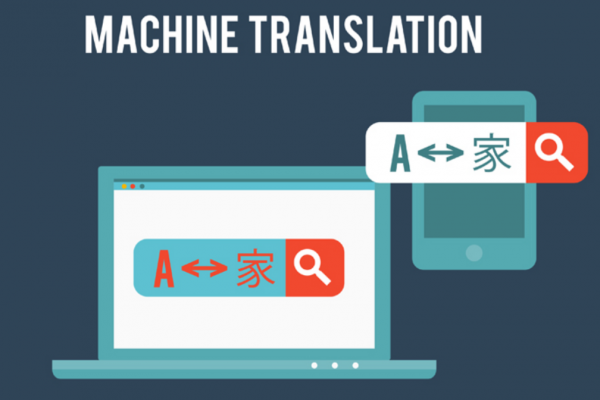
Here are my monthly highlights from paper machine translation and
multilinguality that appeared on arXiv, many of them preprints from the
upcoming EMNLP conference.
Folks from Amazon published a pre-print
that introduces a simple method of how to make pre-trained multilingual
representation more robust towards noisy inputs. It is a very straightforward
approach: they sample typos based on Wikipedia logs and use those during model
training. In addition, they add a contrastive loss that forces the noisy
versions of sentences to get the same representations as the originals.
Aligning word embeddings across languages often fails because the monolingual
word embedding spaces are not isomorphic. In their EMNLP
paper, folks from Johns Hopkins University
try to get rid of this problem by forcing the monolingual embeddings to be
isomorphic