How to Check-Point Deep Learning Models in Keras
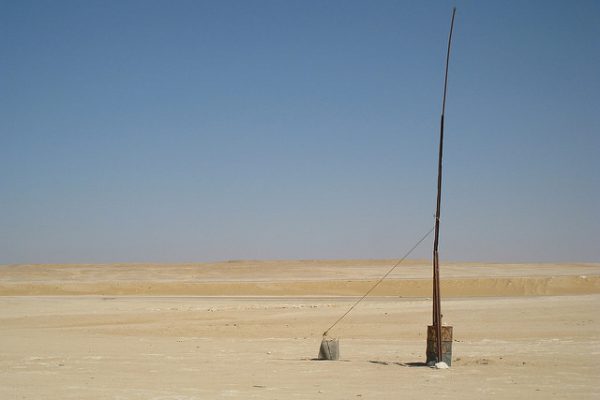
Last Updated on August 27, 2020
Deep learning models can take hours, days or even weeks to train.
If the run is stopped unexpectedly, you can lose a lot of work.
In this post you will discover how you can check-point your deep learning models during training in Python using the Keras library.
Kick-start your project with my new book Deep Learning With Python, including step-by-step tutorials and the Python source code files for all examples.
Let’s get started.
- Update Mar/2017: Updated for Keras 2.0.2, TensorFlow 1.0.1 and Theano 0.9.0.
- Update Mar/2018: Added alternate link to download the dataset.
- Update Sep/2019: Updated for Keras 2.2.5 API.
- Update Oct/2019: Updated for Keras 2.3.0 API.

How to Check-Point Deep Learning Models in Keras
Photo by saragoldsmith, some rights reserved.
Checkpointing Neural Network Models
Application checkpointing is a fault tolerance technique for long running processes.
It is an approach where a snapshot of the state of the system is taken in case of system failure. If there is a problem,
To finish reading, please visit source site