Retrieval Augmentation Reduces Hallucination in Conversation
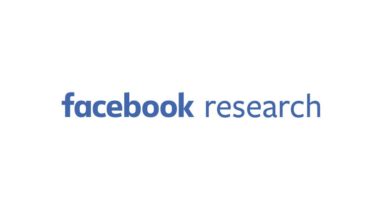
Abstract
Despite showing increasingly human-like conversational abilities, state-of-the-art dialogue models often suffer from factual incorrectness and hallucination of knowledge. In this work we explore the use of neural-retrieval-in-the-loop architectures – recently shown to be effective in open-domain QA – for knowledge-grounded dialogue, a task that is arguably more challenging as it requires querying based on complex multi-turn dialogue context and generating conversationally coherent responses. We study various types of architectures with multiple components – retrievers, rankers, and encoder-decoders – with the goal of maximizing knowledgeability while retaining conversational ability. We demonstrate that our best models obtain state-of-the-art performance on